2022

Medela, Alfonso; Carthy, Taig Mac; Robles, S. Andy Aguilar; Chiesa-Estomba, Carlos M.; Grimalt, Ramon
Automatic SCOring of Atopic Dermatitis Using Deep Learning: A Pilot Study Journal Article
In: JID Innovations, vol. 2, no. 3, pp. 100107, 2022, ISSN: 2667-0267.
@article{MEDELA2022100107,
title = {Automatic SCOring of Atopic Dermatitis Using Deep Learning: A Pilot Study},
author = {Alfonso Medela and Taig Mac Carthy and S. Andy Aguilar Robles and Carlos M. Chiesa-Estomba and Ramon Grimalt},
url = {https://www.sciencedirect.com/science/article/pii/S2667026722000145},
doi = {https://doi.org/10.1016/j.xjidi.2022.100107},
issn = {2667-0267},
year = {2022},
date = {2022-01-01},
urldate = {2022-01-01},
journal = {JID Innovations},
volume = {2},
number = {3},
pages = {100107},
abstract = {Atopic dermatitis (AD) is a chronic, itchy skin condition that affects 15–20% of children but may occur at any age. It is estimated that 16.5 million US adults (7.3%) have AD that initially began at age >2 years, with nearly 40% affected by moderate or severe disease. Therefore, a quantitative measurement that tracks the evolution of AD severity could be extremely useful in assessing patient evolution and therapeutic efficacy. Currently, SCOring Atopic Dermatitis (SCORAD) is the most frequently used measurement tool in clinical practice. However, SCORAD has the following disadvantages: (i) time consuming—calculating SCORAD usually takes about 7–10 minutes per patient, which poses a heavy burden on dermatologists and (ii) inconsistency—owing to the complexity of SCORAD calculation, even well-trained dermatologists could give different scores for the same case. In this study, we introduce the Automatic SCORAD, an automatic version of the SCORAD that deploys state-of-the-art convolutional neural networks that measure AD severity by analyzing skin lesion images. Overall, we have shown that Automatic SCORAD may prove to be a rapid and objective alternative method for the automatic assessment of AD, achieving results comparable with those of human expert assessment while reducing interobserver variability.},
keywords = {},
pubstate = {published},
tppubtype = {article}
}

Chiesa-Estomba, C M; na, M Gra; Medela, A; Sistiaga-Suarez, J A; Lechien, J R; Calvo-Henriquez, C; Mayo-Yanez, M; Vaira, L A; Grammatica, A; Cammaroto, G; Ayad, T; Fagan, J J
Machine Learning Algorithms as a Computer-Assisted Decision Tool for Oral Cancer Prognosis and Management Decisions: A Systematic Review Journal Article
In: ORL, 2022.
@article{Chiesa-Estomba2022-xu,
title = {Machine Learning Algorithms as a Computer-Assisted Decision Tool for Oral Cancer Prognosis and Management Decisions: A Systematic Review},
author = {C M Chiesa-Estomba and M Gra na and A Medela and J A Sistiaga-Suarez and J R Lechien and C Calvo-Henriquez and M Mayo-Yanez and L A Vaira and A Grammatica and G Cammaroto and T Ayad and J J Fagan},
url = {https://www.karger.com/Article/FullText/520672},
year = {2022},
date = {2022-01-01},
urldate = {2022-01-01},
journal = {ORL},
abstract = {Introduction: Despite multiple prognostic indicators described
for oral cavity squamous cell carcinoma (OCSCC), its management
still continues to be a matter of debate. Machine learning is a
subset of artificial intelligence that enables computers to learn
from historical data, gather insights, and make predictions about
new data using the model learned. Therefore, it can be a
potential tool in the field of head and neck cancer. Methods: We
conducted a systematic review. Results: A total of 81 manuscripts
were revised, and 46 studies met the inclusion criteria. Of
these, 38 were excluded for the following reasons: use of a classical statistical method (N = 16), nonspecific for OCSCC (N = 15), and not being related to OCSCC survival (N = 7). In total, 8
studies were included in the final analysis. Conclusions: ML has
the potential to significantly advance research in the field of
OCSCC. Advantages are related to the use and training of ML
models because of their capability to continue training
continuously when more data become available. Future ML research
will allow us to improve and democratize the application of
algorithms to improve the prediction of cancer prognosis and its
management worldwide.},
keywords = {},
pubstate = {published},
tppubtype = {article}
}
for oral cavity squamous cell carcinoma (OCSCC), its management
still continues to be a matter of debate. Machine learning is a
subset of artificial intelligence that enables computers to learn
from historical data, gather insights, and make predictions about
new data using the model learned. Therefore, it can be a
potential tool in the field of head and neck cancer. Methods: We
conducted a systematic review. Results: A total of 81 manuscripts
were revised, and 46 studies met the inclusion criteria. Of
these, 38 were excluded for the following reasons: use of a classical statistical method (N = 16), nonspecific for OCSCC (N = 15), and not being related to OCSCC survival (N = 7). In total, 8
studies were included in the final analysis. Conclusions: ML has
the potential to significantly advance research in the field of
OCSCC. Advantages are related to the use and training of ML
models because of their capability to continue training
continuously when more data become available. Future ML research
will allow us to improve and democratize the application of
algorithms to improve the prediction of cancer prognosis and its
management worldwide.
2021
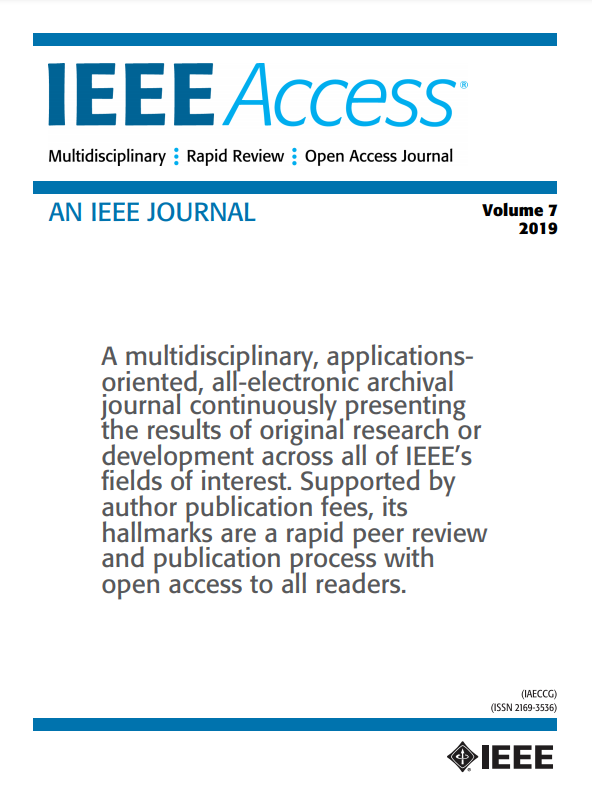
Picon, Artzai; Medela, Alfonso; Sánchez-Peralta, Luisa F.; Cicchi, Riccardo; Bilbao, Roberto; Alfieri, Domenico; Elola, Andoni; Glover, Ben; Saratxaga, Cristina L.
Autofluorescence Image Reconstruction and Virtual Staining for In-Vivo Optical Biopsying Journal Article
In: IEEE Access, vol. 9, pp. 32081-32093, 2021.
@article{9359782,
title = {Autofluorescence Image Reconstruction and Virtual Staining for In-Vivo Optical Biopsying},
author = {Artzai Picon and Alfonso Medela and Luisa F. Sánchez-Peralta and Riccardo Cicchi and Roberto Bilbao and Domenico Alfieri and Andoni Elola and Ben Glover and Cristina L. Saratxaga},
doi = {10.1109/ACCESS.2021.3060926},
year = {2021},
date = {2021-01-01},
urldate = {2021-01-01},
journal = {IEEE Access},
volume = {9},
pages = {32081-32093},
keywords = {},
pubstate = {published},
tppubtype = {article}
}

Chiesa-Estomba, Carlos Miguel; Echaniz, Oier; Suarez, Jon Alexander Sistiaga; González-García, Jose Angel; Larruscain, Ekhiñe; Altuna, Xabier; Medela, Alfonso; Graña, Manuel
Machine Learning Models for Predicting Facial Nerve Palsy in Parotid Gland Surgery for Benign Tumors Journal Article
In: Journal of Surgical Research, vol. 262, pp. 57-64, 2021, ISSN: 0022-4804.
@article{CHIESAESTOMBA202157,
title = {Machine Learning Models for Predicting Facial Nerve Palsy in Parotid Gland Surgery for Benign Tumors},
author = {Carlos Miguel Chiesa-Estomba and Oier Echaniz and Jon Alexander Sistiaga Suarez and Jose Angel González-García and Ekhiñe Larruscain and Xabier Altuna and Alfonso Medela and Manuel Graña},
url = {https://www.sciencedirect.com/science/article/pii/S0022480421000135},
doi = {https://doi.org/10.1016/j.jss.2020.12.053},
issn = {0022-4804},
year = {2021},
date = {2021-01-01},
urldate = {2021-01-01},
journal = {Journal of Surgical Research},
volume = {262},
pages = {57-64},
abstract = {Background
Despite the increasing use of intraoperative facial nerve monitoring during parotid gland surgery (PGS) and the improvement in the preoperative radiological assessment, facial nerve injury (FNI) remains the most severe complication after PGS. Until now, no studies have been published regarding the application of machine learning (ML) for predicting FNI after PGS. We hypothesize that ML would improve the prediction of patients at risk.
Methods
Patients who underwent PGS for benign tumors between June 2010 and June 2019 were included.
Results
Regarding prediction accuracy and performance of each ML algorithm, the K-nearest neighbor and the random forest achieved the highest sensitivity, specificity, positive predictive value, negative predictive value F-score, receiver operating characteristic (ROC)–area under the ROC curve, and accuracy globally. The K-nearest neighbor algorithm achieved performance values above 0.9 for specificity, negative predictive value, F-score and ROC–area under the ROC curve, and the highest sensitivity and positive predictive value.
Conclusions
This study demonstrates that ML prediction models can provide evidence-based predictions about the risk of FNI to otolaryngologists and patients. It is hoped that such algorithms, which use clinical, radiological, histological, and cytological information, can improve the information given to patients before surgery so that they can be better informed of any potential complications.},
keywords = {},
pubstate = {published},
tppubtype = {article}
}
Despite the increasing use of intraoperative facial nerve monitoring during parotid gland surgery (PGS) and the improvement in the preoperative radiological assessment, facial nerve injury (FNI) remains the most severe complication after PGS. Until now, no studies have been published regarding the application of machine learning (ML) for predicting FNI after PGS. We hypothesize that ML would improve the prediction of patients at risk.
Methods
Patients who underwent PGS for benign tumors between June 2010 and June 2019 were included.
Results
Regarding prediction accuracy and performance of each ML algorithm, the K-nearest neighbor and the random forest achieved the highest sensitivity, specificity, positive predictive value, negative predictive value F-score, receiver operating characteristic (ROC)–area under the ROC curve, and accuracy globally. The K-nearest neighbor algorithm achieved performance values above 0.9 for specificity, negative predictive value, F-score and ROC–area under the ROC curve, and the highest sensitivity and positive predictive value.
Conclusions
This study demonstrates that ML prediction models can provide evidence-based predictions about the risk of FNI to otolaryngologists and patients. It is hoped that such algorithms, which use clinical, radiological, histological, and cytological information, can improve the information given to patients before surgery so that they can be better informed of any potential complications.
2020

Medela, Alfonso; Picon, Artzai
Constellation Loss: Improving the Efficiency of Deep Metric Learning Loss Functions for the Optimal Embedding of histopathological images Journal Article
In: J Pathol Inform, vol. 11, pp. 38, 2020.
@article{Medela2020-on,
title = {Constellation Loss: Improving the Efficiency of Deep Metric Learning Loss Functions for the Optimal Embedding of histopathological images},
author = {Alfonso Medela and Artzai Picon},
url = {https://www.jpathinformatics.org/article.asp?issn=2153-3539;year=2020;volume=11;issue=1;spage=38;epage=38;aulast=Medela},
year = {2020},
date = {2020-11-01},
urldate = {2020-11-01},
journal = {J Pathol Inform},
volume = {11},
pages = {38},
abstract = {BACKGROUND: Deep learning diagnostic algorithms are proving
comparable results with human experts in a wide variety of tasks,
and they still require a huge amount of well-annotated data for
training, which is often non affordable. Metric learning
techniques have allowed a reduction in the required annotated
data allowing few-shot learning over deep learning architectures.
AIMS AND OBJECTIVES: In this work, we analyze the
state-of-the-art loss functions such as triplet loss, contrastive
loss, and multi-class N-pair loss for the visual embedding
extraction of hematoxylin and eosin (H&E) microscopy images and
we propose a novel constellation loss function that takes
advantage of the visual distances of the embeddings of the
negative samples and thus, performing a regularization that
increases the quality of the extracted embeddings. MATERIALS AND
METHODS: To this end, we employed the public H&E imaging dataset
from the University Medical Center Mannheim (Germany) that
contains tissue samples from low-grade and high-grade primary
tumors of digitalized colorectal cancer tissue slides. These
samples are divided into eight different textures (1. tumour
epithelium, 2. simple stroma, 3. complex stroma, 4. immune cells,
5. debris and mucus, 6. mucosal glands, 7. adipose tissue and 8.
background,). The dataset was divided randomly into train and
test splits and the training split was used to train a classifier
to distinguish among the different textures with just 20 training
images. The process was repeated 10 times for each loss function.
Performance was compared both for cluster compactness and for
classification accuracy on separating the aforementioned
textures. RESULTS: Our results show that the proposed loss
function outperforms the other methods by obtaining more compact
clusters (Davis-Boulding: 1.41 $pm$ 0.08, Silhouette: 0.37 $pm$
0.02) and better classification capabilities (accuracy: 85.0
$pm$ 0.6) over H and E microscopy images. We demonstrate that
the proposed constellation loss can be successfully used in the
medical domain in situations of data scarcity.},
keywords = {},
pubstate = {published},
tppubtype = {article}
}
comparable results with human experts in a wide variety of tasks,
and they still require a huge amount of well-annotated data for
training, which is often non affordable. Metric learning
techniques have allowed a reduction in the required annotated
data allowing few-shot learning over deep learning architectures.
AIMS AND OBJECTIVES: In this work, we analyze the
state-of-the-art loss functions such as triplet loss, contrastive
loss, and multi-class N-pair loss for the visual embedding
extraction of hematoxylin and eosin (H&E) microscopy images and
we propose a novel constellation loss function that takes
advantage of the visual distances of the embeddings of the
negative samples and thus, performing a regularization that
increases the quality of the extracted embeddings. MATERIALS AND
METHODS: To this end, we employed the public H&E imaging dataset
from the University Medical Center Mannheim (Germany) that
contains tissue samples from low-grade and high-grade primary
tumors of digitalized colorectal cancer tissue slides. These
samples are divided into eight different textures (1. tumour
epithelium, 2. simple stroma, 3. complex stroma, 4. immune cells,
5. debris and mucus, 6. mucosal glands, 7. adipose tissue and 8.
background,). The dataset was divided randomly into train and
test splits and the training split was used to train a classifier
to distinguish among the different textures with just 20 training
images. The process was repeated 10 times for each loss function.
Performance was compared both for cluster compactness and for
classification accuracy on separating the aforementioned
textures. RESULTS: Our results show that the proposed loss
function outperforms the other methods by obtaining more compact
clusters (Davis-Boulding: 1.41 $pm$ 0.08, Silhouette: 0.37 $pm$
0.02) and better classification capabilities (accuracy: 85.0
$pm$ 0.6) over H and E microscopy images. We demonstrate that
the proposed constellation loss can be successfully used in the
medical domain in situations of data scarcity.

Argüeso, David; Picon, Artzai; Irusta, Unai; Medela, Alfonso; San-Emeterio, Miguel G; Bereciartua, Arantza; Alvarez-Gila, Aitor
Few-Shot Learning approach for plant disease classification using images taken in the field Journal Article
In: Computers and Electronics in Agriculture, vol. 175, pp. 105542, 2020, ISSN: 0168-1699.
@article{ARGUESO2020105542,
title = {Few-Shot Learning approach for plant disease classification using images taken in the field},
author = {David Argüeso and Artzai Picon and Unai Irusta and Alfonso Medela and Miguel G San-Emeterio and Arantza Bereciartua and Aitor Alvarez-Gila},
url = {https://www.sciencedirect.com/science/article/pii/S0168169920302544},
doi = {https://doi.org/10.1016/j.compag.2020.105542},
issn = {0168-1699},
year = {2020},
date = {2020-01-01},
urldate = {2020-01-01},
journal = {Computers and Electronics in Agriculture},
volume = {175},
pages = {105542},
abstract = {Prompt plant disease detection is critical to prevent plagues and to mitigate their effects on crops. The most accurate automatic algorithms for plant disease identification using plant field images are based on deep learning. These methods require the acquisition and annotation of large image datasets, which is frequently technically or economically unfeasible. This study introduces Few-Shot Learning (FSL) algorithms for plant leaf classification using deep learning with small datasets. For the study 54,303 labeled images from the PlantVillage dataset were used, comprising 38 plant leaf and/or disease types (classes). The data was split into a source (32 classes) and a target (6 classes) domain. The Inception V3 network was fine-tuned in the source domain to learn general plant leaf characteristics. This knowledge was transferred to the target domain to learn new leaf types from few images. FSL using Siamese networks and Triplet loss was used and compared to classical fine-tuning transfer learning. The source and target domain sets were split into a training set (80%) to develop the methods and a test set (20%) to obtain the results. Algorithm performance was evaluated using the total accuracy, and the precision and recall per class. For the FSL experiments the algorithms were trained with different numbers of images per class and the experiments were repeated 20 times to statistically characterize the results. The accuracy in the source domain was 91.4% (32 classes), with a median precision/recall per class of 93.8%/92.6%. The accuracy in the target domain was 94.0% (6 classes) learning from all the training data, and the median accuracy (90% confidence interval) learning from 1 image per class was 55.5 (46.0–61.7)%. Median accuracies of 80.0 (76.4–86.5)% and 90.0 (86.1–94.2)% were reached for 15 and 80 images per class, yielding a reduction of 89.1% (80 images/class) in the training dataset with only a 4-point loss in accuracy. The FSL method outperformed the classical fine tuning transfer learning which had accuracies of 18.0 (16.0–24.0)% and 72.0 (68.0–77.3)% for 1 and 80 images per class, respectively. It is possible to learn new plant leaf and disease types with very small datasets using deep learning Siamese networks with Triplet loss, achieving almost a 90% reduction in training data needs and outperforming classical learning techniques for small training sets.},
keywords = {},
pubstate = {published},
tppubtype = {article}
}
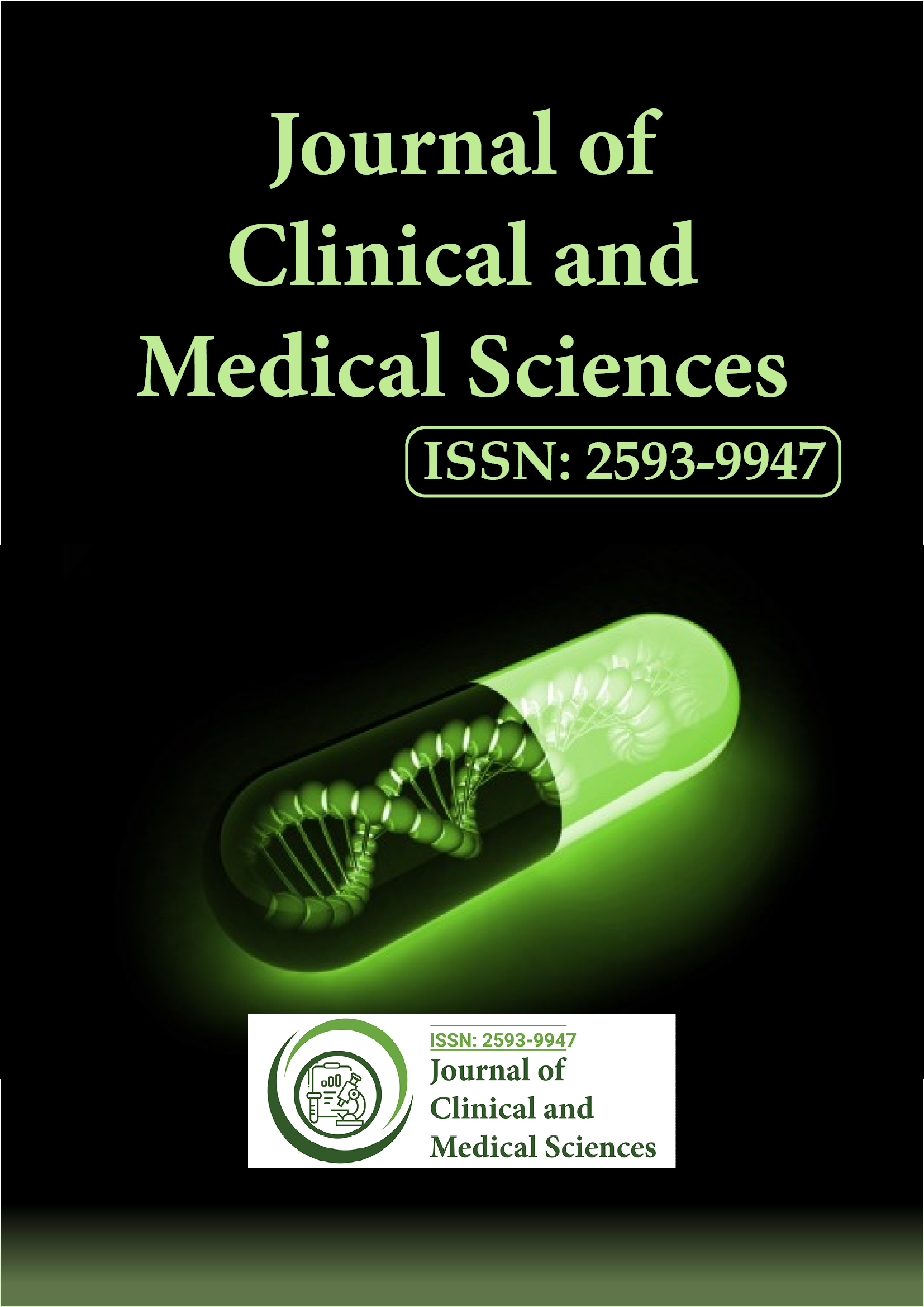
Chiesa-Estomba, Carlos M; Sistiaga-Suarez, Jon A; González-Garc'ia, José Ángel; ne Larruscain, Ekhi; Cammaroto, Giovanni; Mayo-Yánez, Miguel; Lechien, Jerome R; Calvo-Henr'iquez, Christian; Altuna, Xabier; Medela, Alfonso
Artificial Neural Network as a Tool to Predict Facial Nerve Palsy in Parotid Gland Surgery for Benign Tumors Journal Article
In: Medical Sciences, vol. 8, no. 4, pp. 42, 2020.
@article{Chiesa-Estomba2020-fm,
title = {Artificial Neural Network as a Tool to Predict Facial Nerve Palsy in Parotid Gland Surgery for Benign Tumors},
author = {Carlos M Chiesa-Estomba and Jon A Sistiaga-Suarez and José Ángel González-Garc'ia and Ekhi ne Larruscain and Giovanni Cammaroto and Miguel Mayo-Yánez and Jerome R Lechien and Christian Calvo-Henr'iquez and Xabier Altuna and Alfonso Medela},
url = {https://pubmed.ncbi.nlm.nih.gov/33036481/},
year = {2020},
date = {2020-01-01},
urldate = {2020-01-01},
journal = {Medical Sciences},
volume = {8},
number = {4},
pages = {42},
keywords = {},
pubstate = {published},
tppubtype = {article}
}
2019

Medela, Alfonso; Picon, Artzai; Saratxaga, Cristina L.; Belar, Oihana; Cabezón, Virginia; Cicchi, Riccardo; Bilbao, Roberto; Glover, Ben
Few Shot Learning in Histopathological Images: Reducing the Need of Labeled Data on Biological Datasets Inproceedings
In: 2019 IEEE 16th International Symposium on Biomedical Imaging (ISBI 2019), pp. 1860-1864, 2019.
@inproceedings{8759182,
title = {Few Shot Learning in Histopathological Images: Reducing the Need of Labeled Data on Biological Datasets},
author = {Alfonso Medela and Artzai Picon and Cristina L. Saratxaga and Oihana Belar and Virginia Cabezón and Riccardo Cicchi and Roberto Bilbao and Ben Glover},
doi = {10.1109/ISBI.2019.8759182},
year = {2019},
date = {2019-01-01},
urldate = {2019-01-01},
booktitle = {2019 IEEE 16th International Symposium on Biomedical Imaging (ISBI 2019)},
pages = {1860-1864},
keywords = {},
pubstate = {published},
tppubtype = {inproceedings}
}